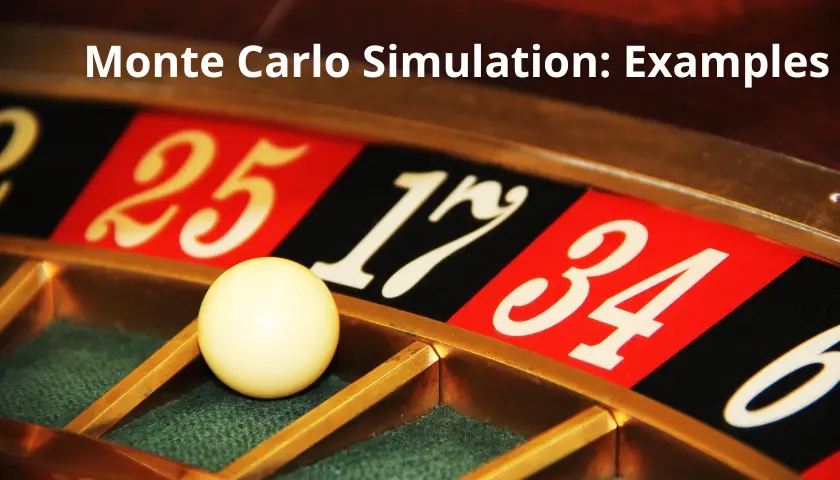
Monte Carlo Simulation: Examples
EXECUTIVE SUMMARY
A Monte Carlo simulation uses samples of input data together with a known mathematical model to predict probabilistic outcomes. The model we use in our publication is geometrical Brownian motion or GBM. GBM is widely used to model many financial variables such as changes in stock prices, company’s EBITDA, revenue, etc.
GBM is a probabilistic model in the sense that it includes an element of uncertainty or randomness in its prediction. Specifically, included in GBM formular are randomly generated numbers following normal distribution with a mean of 0 and a standard deviation of 1.
Generally, Monte Carlo simulation is used in cases involving path dependency, i.e., situations where the result in a subsequent period depends on the results in a prior period.
When you use a probabilistic model to simulate an outcome, you will get different results for each trial. Runing enough simulations to produce different outcomes will mimic real-life results. Using probabilistic models contrasts conventional forecasting methods which produce more deterministic results. In our case we ran 10,000 trials in Microsoft Excel to determine the expected sough-after value.
Our illustration of Monte Carlo simulation includes three examples. As part of first example, we determine fair value of a contingent consideration payable in connection with acquisition of a business. Second example presents an employee bonus plan with terms similar to contingent consideration from first example. Last example has to do with determination of a bonus liability, dependent on changes in company’s stock price. All our examples cover Monte Carlo simulation to estimate fair values for financial reporting purposes.
As part of our analysis, we explain key concepts used in GBM valuation, including risk-neutral valuation, use of randomly generated number following normal distribution pattern. Our publication also includes detailed step by step application of GBM formula.